
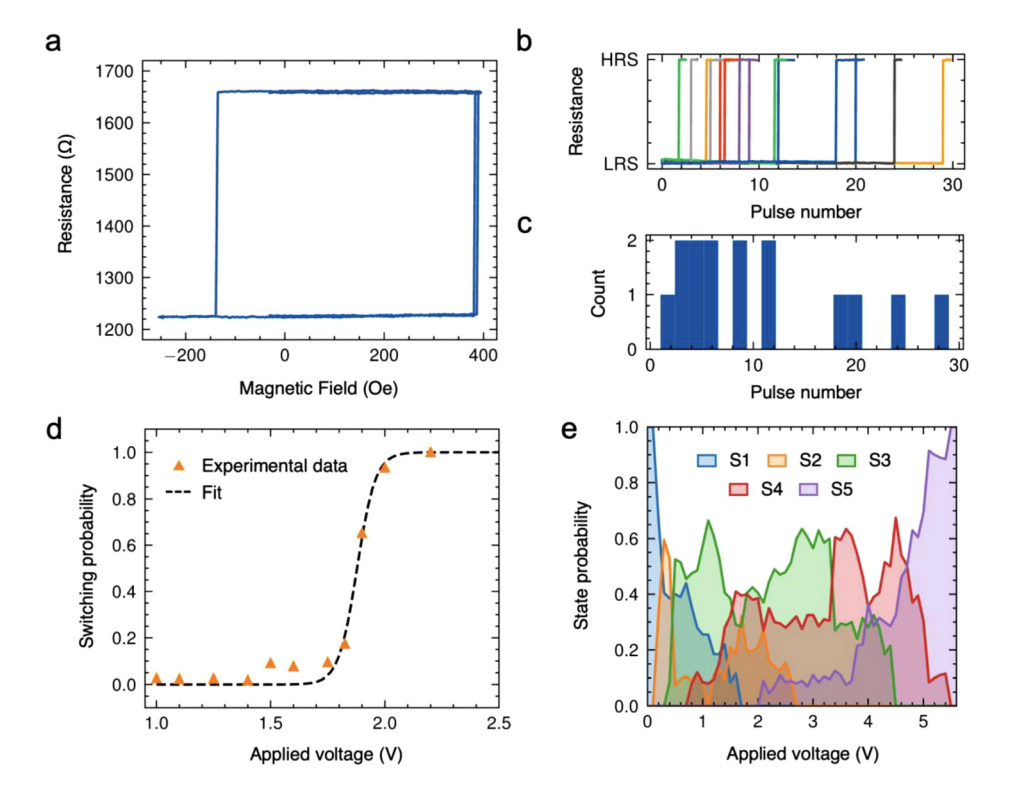
The spatiotemporal nature of neuronal behavior in spiking neural networks (SNNs) makes SNNs promising for edge applications that require high energy efficiency. To realize SNNs in hardware, spintronic neuron implementations can bring advantages of scalability and energy efficiency. Domain wall (DW)-based magnetic tunnel junction (MTJ) devices are well suited for probabilistic neural networks given their intrinsic integrate-and-fire behavior with tunable stochasticity. Here, we present a scaled DW-MTJ neuron with voltage-dependent firing probability. The measured behavior was used to simulate a SNN that attains accuracy during learning compared to an equivalent, but more complicated, multi-weight DW-MTJ device. The validation accuracy during training was also shown to be comparable to an ideal leaky integrate and fire device. However, during inference, the binary DW-MTJ neuron outperformed the other devices after Gaussian noise was introduced to the Fashion-MNIST classification task. This work shows that DW-MTJ devices can be used to construct noise-resilient networks suitable for neuromorphic computing on the edge.
Find the link here: https://doi.org/10.1063/5.0152211

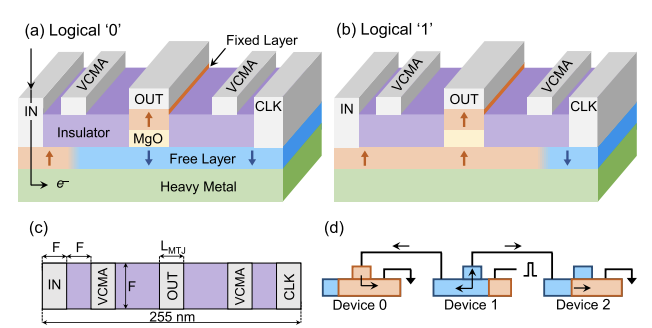
The domain wall-magnetic tunnel junction (DW-MTJ) is a versatile device that can simultaneously store data and perform computations. These three-terminal devices are promising for digital logic due to their nonvolatility, low-energy operation, and radiation hardness. Here, we augment the DW-MTJ logic gate with voltage-controlled magnetic anisotropy (VCMA) to improve the reliability of logical concatenation in the presence of realistic process variations. VCMA creates potential wells that allow for reliable and repeatable localization of domain walls (DWs). The DW-MTJ logic gate supports different fanouts, allowing for multiple inputs and outputs for a single device without affecting the area. We simulate a systolic array of DW-MTJ multiply-accumulate (MAC) units with 4-bit and 8-bit precision, which uses the nonvolatility of DW-MTJ logic gates to enable fine-grained pipelining and high parallelism. The DW-MTJ systolic array provides comparable throughput and efficiency to state-of-the-art CMOS systolic arrays while being radiation-hard. These results improve the feasibility of using DW-based processors, especially for extreme environment applications such as space.
Find the link here: https://doi.org/10.1109/JXCDC.2023.3266441
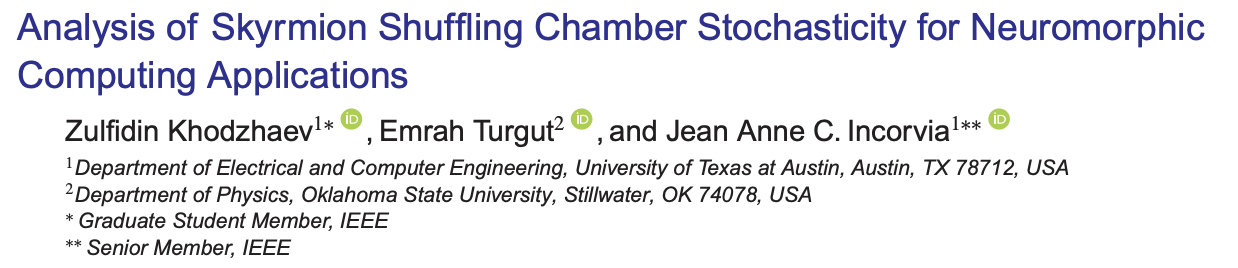
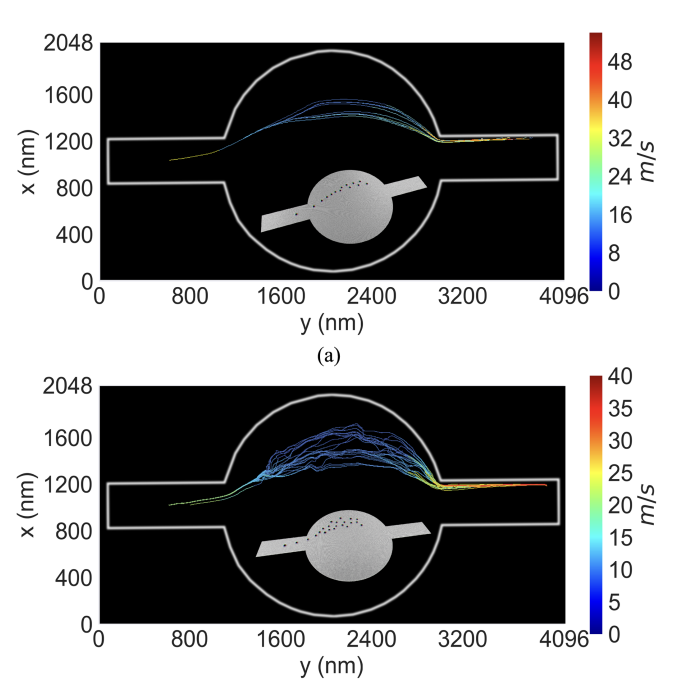
In this study, micromagnetic simulations of a magnetic skyrmion reshuffling chamber for probabilistic computing applications are performed.The skyrmion shuffling chamber is modeled with a custom current density masking technique to capture current density variation, grain boundary variations, and anisotropy changes. The results show that the skyrmion oscillatory dynamics contribute to the system’s stochasticity, allowing uncorrelated signals to be achieved with a single chamber. Our findings indicate that uncorrelated signals are generally achieved at all temperatures simulated, with the skyrmion diameter playing a role in the resulting stochasticity. Furthermore, we find that local temperature control has the benefit of not affecting the overall skyrmion diameter, while still perturbing the skyrmion trajectory. The results from varying chamber size, global temperature, and local temperature are analyzed using Pearson correlation coefficient and p-value. This research contributes to the development of tunable probabilistic computing devices and artificial synapses using magnetic skyrmions.
Find the link here: https://doi.org/10.1109/LMAG.2023.3280120
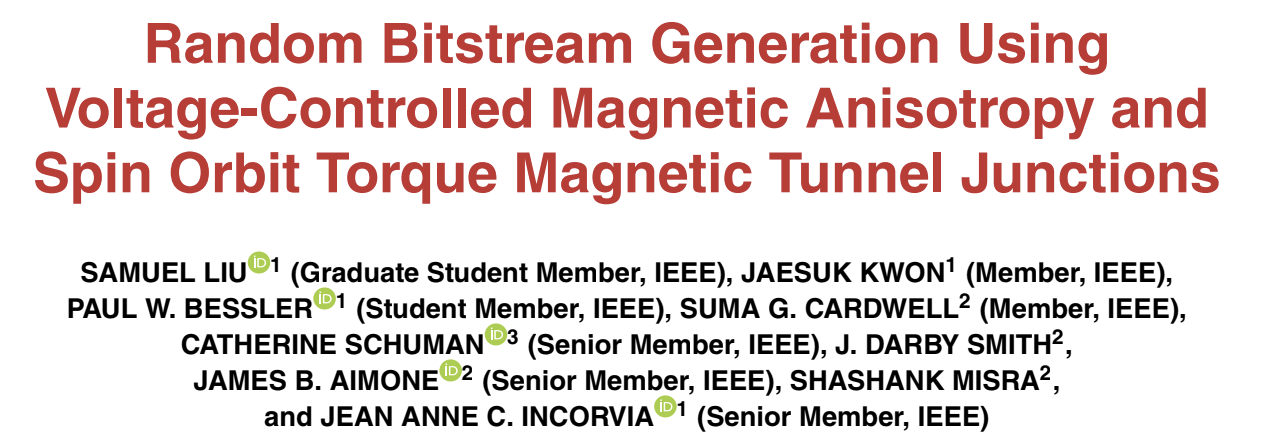
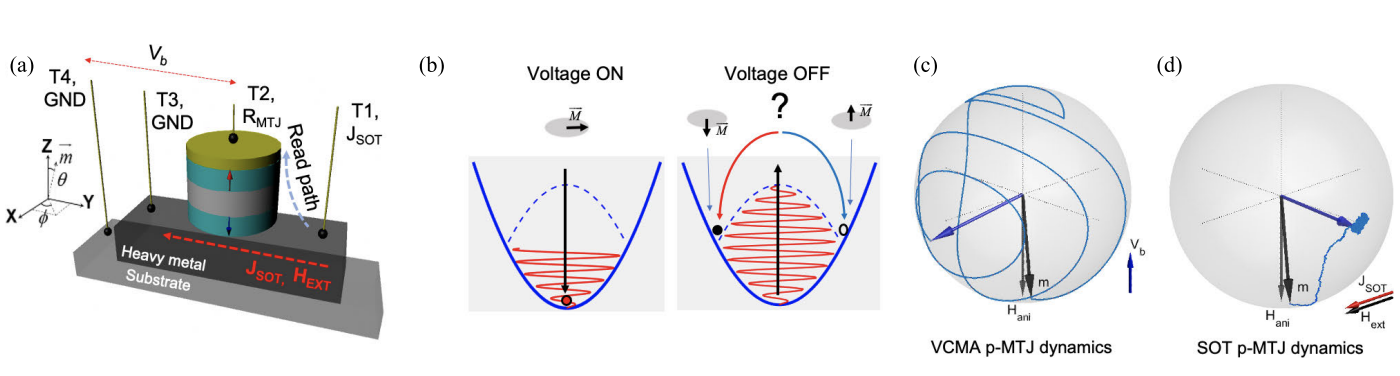
Probabilistic computing using random number generators (RNGs) can leverage the inherent stochasticity of nanodevices for system-level benefits. Device candidates for this application need to produce highly random ‘‘coinflips’’ while also having tunable biasing of the coin. The magnetic tunnel junction (MTJ) has been studied as an RNG due to its thermally-driven magnetization dynamics, often using spin transfer torque (STT) current amplitude to control the random switching of the MTJ free layer (FL) magnetization, here called the stochastic write method. There are additional knobs to control the MTJ- RNG, including voltage-controlled magnetic anisotropy (VCMA) and spin orbit torque (SOT), and there is a need to systematically study and compare these methods. We build an analytical model of the MTJ to characterize using VCMA and SOT to generate random bit streams. The results show that both methods produce high-quality, uniformly distributed bitstreams. Biasing the bitstreams using either STT current or an applied magnetic field shows a sigmoidal distribution versus bias amplitude for both VCMA and SOT, compared to less sigmoidal for stochastic write. The energy consumption per sample is calculated to be 0.1 pJ (SOT), 1 pJ (stochastic write), and 20 pJ (VCMA), revealing the potential energy benefit of using SOT and showing using VCMA may require higher damping materials. The generated bitstreams are then applied to two tasks: generating an arbitrary probability distribution and using the MTJ-RNGs as stochastic neurons to perform simulated annealing, where both VCMA and SOT methods show the ability to effectively minimize the system energy with a small delay and low energy. These results show the flexibility of the MTJ as a true RNG and elucidate design parameters for optimizing the device operation for applications.
Find the link here: https://doi.org/10.1109/JXCDC.2022.3231550,
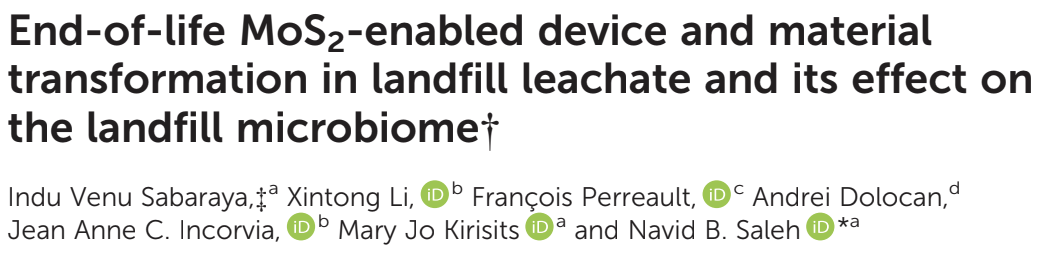
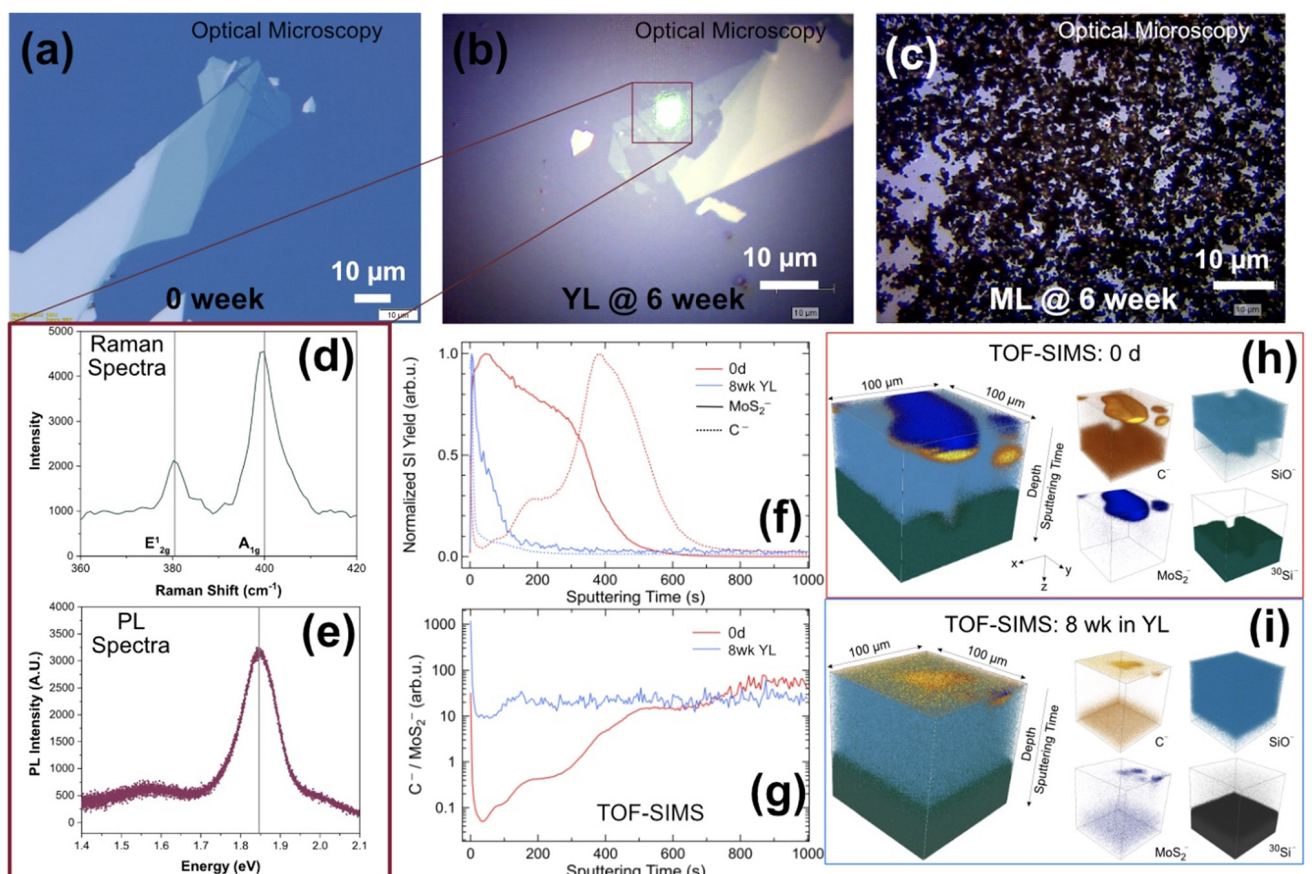
The safety of nano-enabled devices must be assessed throughout the device lifecycle. Landfills are the end-of-life repository for functional devices; however, the dynamism of leachate age and complexity of leachate chemistry make material safety assessments challenging. This study evaluates the transformation and effects of water treatment membranes and transistors enabled with MoS2—a transition metal dichalcogenide (TMD) used in electronic transistors and futuristic wearable electronics—in complex landfill conditions. Device decay and MoS2 transformation are studied with a suite of characterization tools. The effects of MoS2 colloids on microbial diversity in young and mature landfill leachates are investigated. Results indicate that MoS2-enabled membranes and transistors become attached to residues from the leachate but do not undergo major chemical decay. Furthermore, complex environments like leachates are robust, resisting microbiome changes upon exposure to MoS2. The results obtained under the specific study conditions indicate that disposal of MoS2-enabled devices in landfills does not induce significant deleterious effects on the landfill in the absence of photo-transformation (as is the case in covered landfills). However, further studies are needed to assess whether a high concentration of MoS2, a likely result of its accumulation in the landfill over time, has the potential to substantially change the leachate microbiome.
Find the link here: https://doi.org/10.1039/d2en00707j

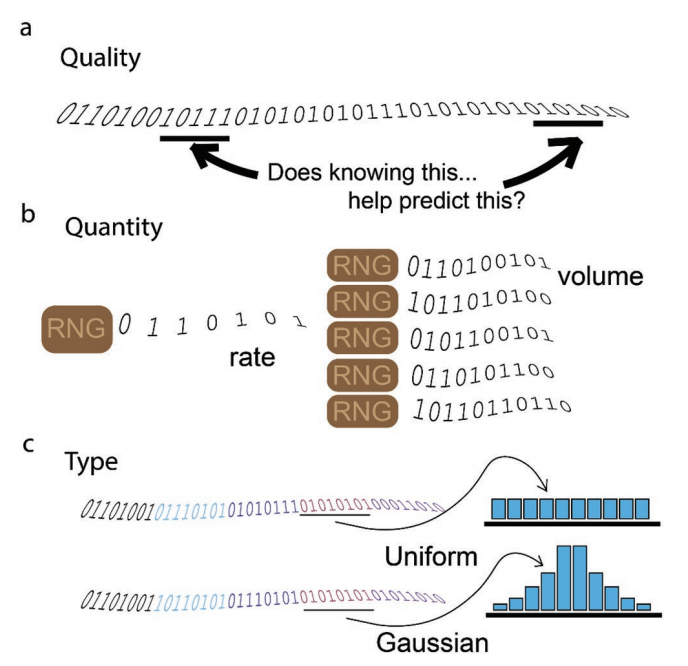
The brain has effectively proven a powerful inspiration for the development of computing architectures in which processing is tightly integrated with memory, communication is event-driven, and analog computation can be performed at scale. These neuromorphic systems increasingly show an ability to improve the efficiency and speed of scientific computing and artificial intelligence applications. Herein, it is proposed that the brain’s ubiquitous stochasticity represents an additional source of inspiration for expanding the reach of neuromorphic computing to probabilistic applications. To date, many efforts exploring probabilistic computing have focused primarily on one scale of the microelectronics stack, such as implementing probabilistic algorithms on deterministic hardware or developing probabilistic devices and circuits with the expectation that they will be leveraged by eventual probabilistic architectures. A co-design vision is described by which large numbers of devices, such as magnetic tunnel junctions and tunnel diodes, can be operated in a stochastic regime and incorporated into a scalable neuromorphic architecture that can impact a number of probabilistic computing applications, such as Monte Carlo simulations and Bayesian neural networks. Finally, a framework is presented to categorize increasingly advanced hardware-based probabilistic computing technologies.
Find the link here: https://doi.org/10.1002/adma.202204569
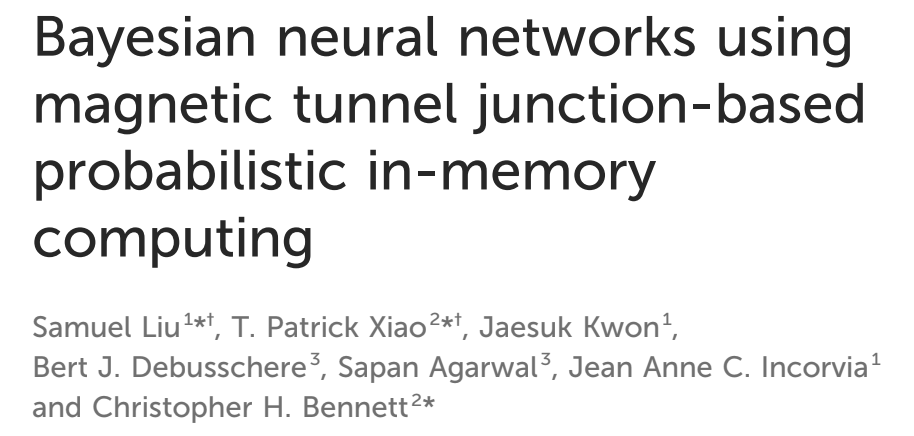
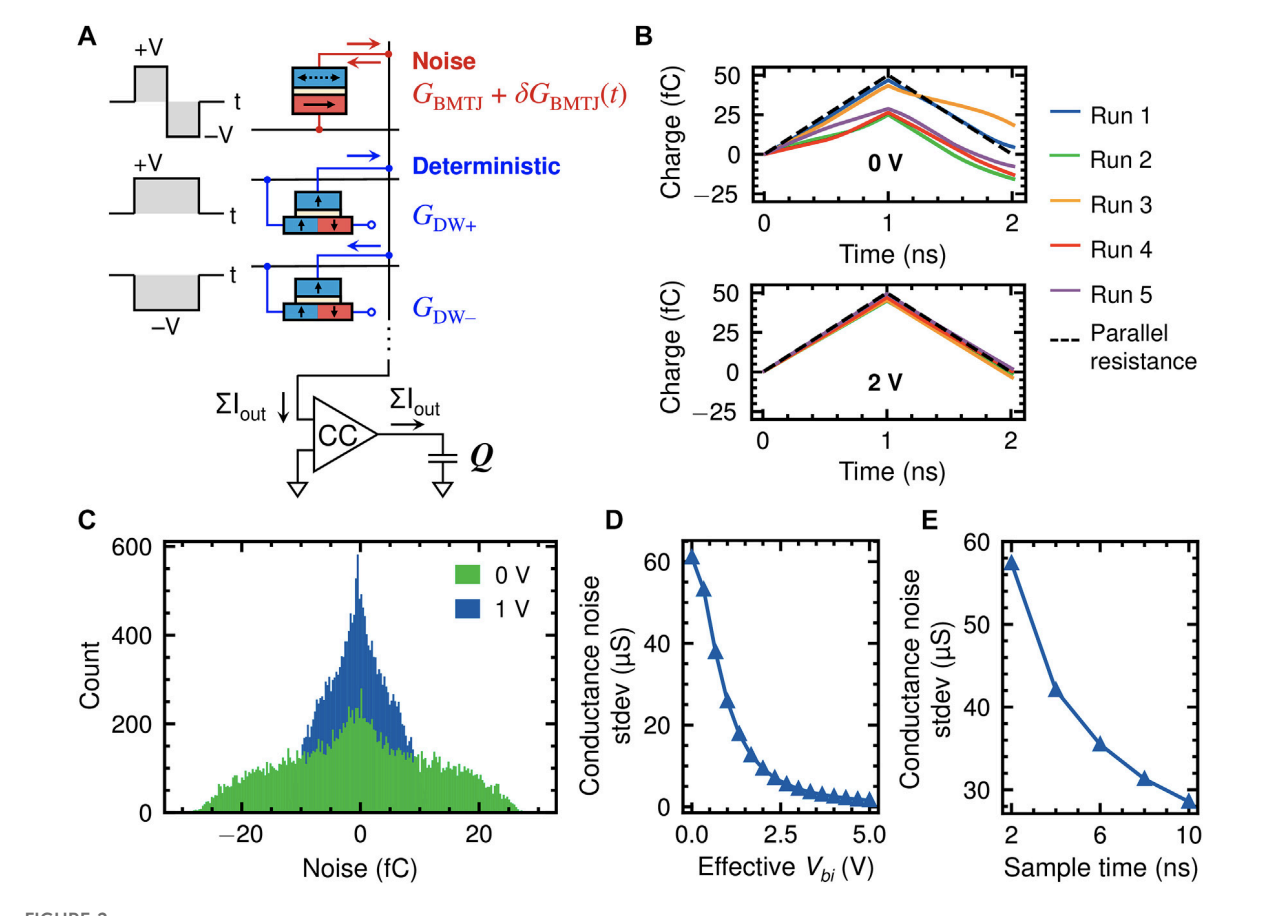
Bayesian neural networks (BNNs) combine the generalizability of deep neural networks (DNNs) with a rigorous quantification of predictive uncertainty, which mitigates overfitting and makes them valuable for high-reliability or safety-critical applications. However, the probabilistic nature of BNNs makes them more computationally intensive on digital hardware and so far, less directly amenable to acceleration by analog in-memory computing as compared to DNNs. This work exploits a novel spintronic bit cell that efficiently and compactly implements Gaussian-distributed BNN values. Specifically, the bit cell combines a tunable stochastic magnetic tunnel junction (MTJ) encoding the trained standard deviation and a multi-bit domain-wall MTJ device independently encoding the trained mean. The two devices can be integrated within the same array, enabling highly efficient, fully analog, probabilistic matrix-vector multiplications. We use micromagnetics simulations as the basis of a system-level model of the spintronic BNN accelerator, demonstrating that our design yields accurate, well-calibrated uncertainty estimates for both classification and regression problems and matches software BNN performance. This result paves the way to spintronic in-memory computing systems implementing trusted neural networks at a modest energy budget.
Find the link here: https://doi.org/10.3389/fnano.2022.1021943

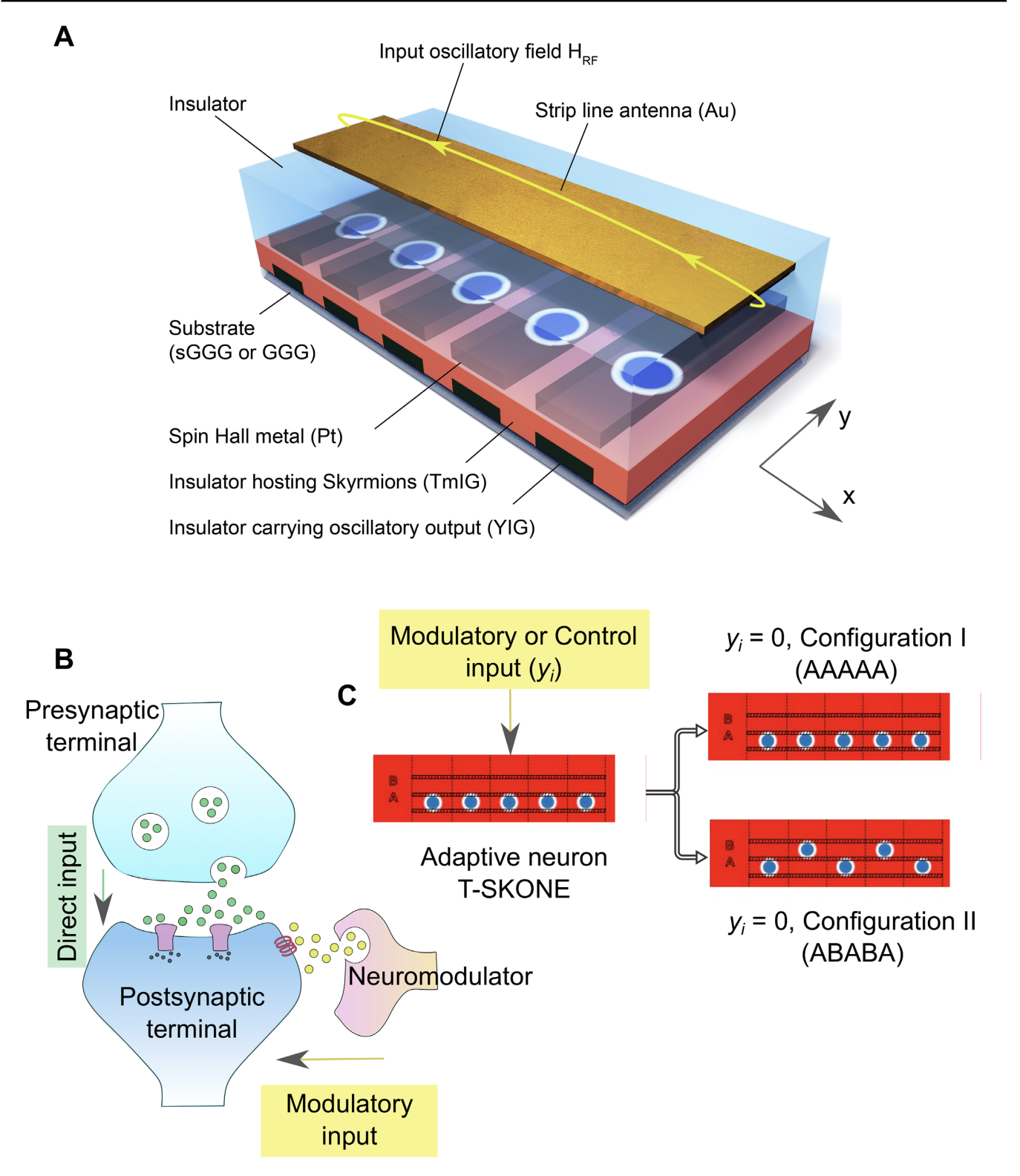
Neuromorphic computing mimics the organizational principles of the brain in its quest to replicate the brain’s intellectual abilities. An impressive ability of the brain is its adaptive intelligence, which allows the brain to regulate its functions “on the fly” to cope with myriad and ever-changing situations. In particular, the brain displays three adaptive and advanced intelligence abilities of context-awareness, cross frequency coupling, and feature binding. To mimic these adaptive cognitive abilities, we design and simulate a novel, hardware-based adaptive oscillatory neuron using a lattice of magnetic skyrmions. Charge current fed to the neuron reconfigures the skyrmion lattice, thereby modulating the neuron’s state, its dynamics and its transfer function “on the fly.” This adaptive neuron is used to demonstrate the three cognitive abilities, of which context-awareness and cross-frequency coupling have not been previously realized in hardware neurons. Additionally, the neuron is used to construct an adaptive artificial neural network (ANN) and perform context-aware diagnosis of breast cancer. Simulations show that the adaptive ANN diagnoses cancer with higher accuracy while learning faster and using a more compact and energy-efficient network than a nonadaptive ANN. The work further describes how hardware-based adaptive neurons can mitigate several critical challenges facing contemporary ANNs. Modern ANNs require large amounts of training data, energy, and chip area, and are highly task-specific; conversely, hardware-based ANNs built with adaptive neurons show faster learning, compact architectures, energy-efficiency, fault-tolerance, and can lead to the realization of broader artificial intelligence.
Find the link here: https://doi.org/10.1093/pnasnexus/pgac206
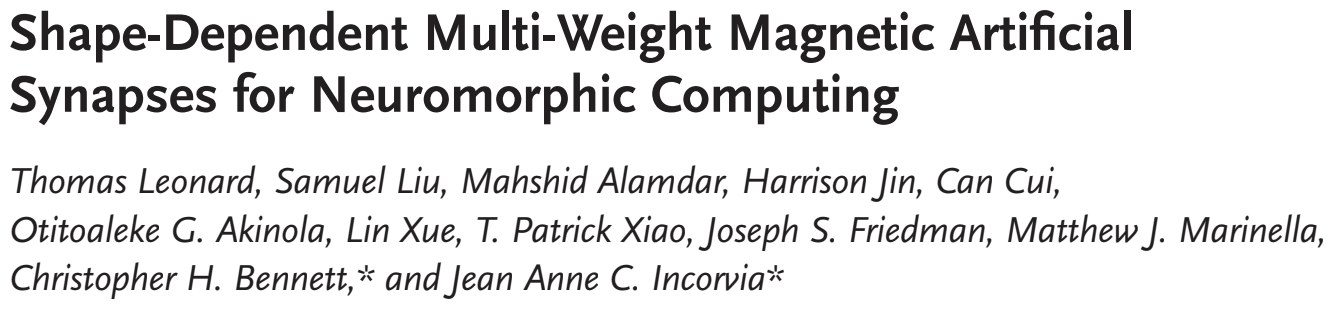
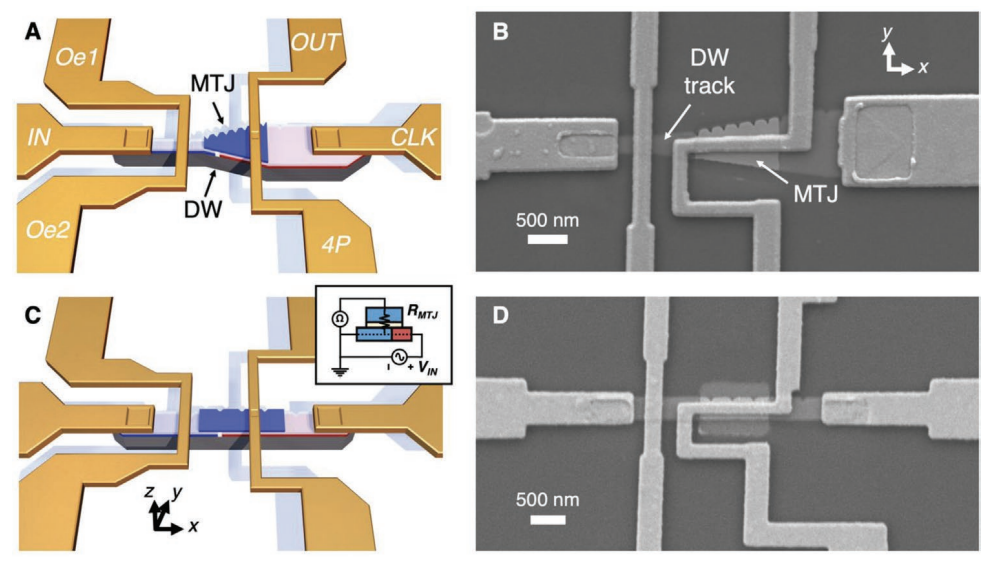